Systemic Racism Measures
Research on racism and health has focused on personal experiences of being the target of racial discrimination. There is abundant evidence that this is an important health-damaging determinant of racial inequities in health. It is also important to study other facets of racism, including systemic racism.
Systemic racism has been conceptualized in various ways, but generally has been used to describe systems, institutions, and structures beyond individual-level practices that reinforce white supremacy. The term “systemic racism” has often been used interchangeably with “structural racism” and “institutional racism”; however, some have noted important distinctions (1). Institutional racism is sometimes considered to be an aspect of structural racism, referring to “discriminatory policies and practices carried out within and between state or non-state institutions on the basis of racialized group membership” (2) by meso-level actors which prevent nonwhites from accessing goods, services, and opportunities (3, 4). It is distinguished from individual/interpersonal experiences of racism, focusing on practices and policies in institutional settings. Structural racism, which tends to be used more synonymously with systemic racism, emphasizes how power works in tandem across institutions and societal levels and are interconnected to enable a dominating force that permeates all aspects of society, with the result being the maintenance of white supremacy, and the undermining of nonwhites (4–7). Some distinguish systemic racism by its somewhat greater emphasis on history and its role in shaping contemporary social and political systems (8, 9). For example, redlining—the devaluation of houses in Black neighborhoods—resulted in poorer quality education and lower graduation rates stemming from schools in those areas having a lower tax base; this led to concentrated poverty, crime, and the disproportionate incarceration of Black Americans (10). The consequences of redlining are still apparent today, with redlined neighborhoods having fewer health resources and greater barriers to upward mobility (3, 11, 12).
Systemic racism has been indexed using a wide range of measures that have been used to proxy salient domains in which racism operates. The examples below are not an exhaustive list; rather, they are meant to illustrate some ways in which indicators of systemic racism may be incorporated in research on racial inequities in health. We hope that this entry will stimulate increased use of these measures, which are derived primarily from administrative data; we believe that when used in tandem with self-reported measures of racism, their utility can be mutually enhanced.
-
Residential segregation. Residential segregation is often considered an indicator of systemic racism in population health research, and includes dimensions of: (1) evenness, which has been operationalized using the dissimilarity index, entropy index, and the divergence index; (2) exposure and isolation (e.g., interaction index, isolation index); (3) concentration or density (e.g., Duncan’s delta index, absolute concentration index, relative concentration index); and clustering, or the degree to which neighborhoods that are predominantly of one group are adjacent to neighborhoods that are also comprised of the same group (e.g., absolute clustering index, distance-decay interaction index) (13). An increasingly used measure of residential segregation is the Index of Concentration at the Extremes (ICE), used to measure the degree of polarization between two groups (14). Measures of residential conditions also highlight structural inequities along racial lines. These include measures of the built and service environment (e.g., objective indicators of neighborhood quality, sidewalks, parks, access to healthcare, supermarkets, and other outlets) (15, 16); as well as indicators of the physical environment (e.g., air pollution, water quality), which have shown strong associations with racial residential segregation (17, 18).
-
Housing. Systemic racism in housing markets has been examined using the Home Mortgage and Disclosure Act (HMDA) database, which has been used to measure lending discrimination (19). The Racial Bias in Mortgage Lending Index calculates the adjusted odds of home mortgage application denial for a Black vs. white applicant in a geographical area (20). The Redlining Index calculates the odds of denial of a mortgage application in a particular area compared to another area (21).
-
Policing. Police killings and nonfatal police violence have been used as an indicator of systemic racism (22). Two databases that have been used in this research are the Mapping Police Violence database (MPVD) which is sourced from state police databases and combined with data from the Fatal Encounters database (23); and the Fatal Encounters database which is a crowdsourced, citizen-science initiative that tracks police killings (24). Other research on police violence has used data from the Guardian’s ‘The Counted” and the Washington Post’s “Fatal Force” database (25, 26). Finally, others have utilized information regarding neighborhood-level aggressive policing (i.e., frisking and use of force by police) and its impact on health (27).
-
Incarceration. Incarceration data is increasingly being utilized as an indicator of systemic racism; however, there are no established measures (28, 29). Studies have calculated current and former rates of incarceration by race using data from the U.S. Department of Justice and U.S. Bureau of Prisons (29, 30). Others have leveraged data from Public Use Microdata Samples, collected via the decennial census which includes data on the institutionalized population (31, 32). Recently available data from the Vera Institute of Justice includes national jail and prison incarceration rates (33). Other have also used data from the Justice Atlas of Sentencing and Corrections to examine the impact of living in high-incarceration neighborhoods on health (34). Data on rates of capital punishment and other judicial treatment is available through the Death Penalty Information Center and Bureau of Justice Statistics (35, 36).
-
Employment. Systemic racism in hiring has been assessed through field and audit studies (37). One large field study was conducted involving 83,000 fictitious applications in response to jobs posted by 108 of the largest U.S. employers (38). Another measure of racism in employment is the rate of employment discrimination complaints filed with the Equal Employment Opportunity Commission (EEOC) (39). A similar publicly available state-level dataset can be obtained through the Center for Public Integrity (40). Data from the U.S. Census Bureau and American Community Survey have been used to calculate Black-white ratios in educational attainment (proportion of the population ³[CKW1] 25 with a Bachelor’s degree or higher), employment (proportion of the civilian labor force not currently employed), and occupation status (proportion of the employed civilians in professional, executive, or managerial positions) (41).
-
Healthcare. There are several measures of systemic racism in healthcare and medical contexts. For example, data from the National Hospital Ambulatory Care Survey (NHAMCS) has been used to examine mean emergency department wait times (42). Other datasets that have been leveraged include the American Time Use Survey (ATUS) and the Scientific Registry of Transplant Recipients database (43, 44). Others have assessed racism in healthcare through field and audit studies (45, 46). Racial bias in medical algorithms has also been used as an indicator of systemic racism in healthcare (47).
-
Political Participation. Systemic racism has also been assessed as the Black-white ratio in political processes and participation (48). Data from the U.S. Census Bureau can be used to calculate the proportion of individuals registered to vote and those who voted in recent elections by race (49). The relative proportion of Black to white state elected officials has also been examined using data from the National Conference of State Legislatures (50). Data is also available through the Cooperative Congressional Election Study and RAND Corporation on barriers to voting, including wait times and state voting laws (51, 52).
We encourage researchers to incorporate measures of systemic racism in their research. The selection of measures should be guided by the relevant unit of analysis that is being studied (e.g., scale, geography), in addition to conceptual considerations (e.g., based on study population, outcomes). Through increased use of these measures, research on racism and health can better elucidate the mechanisms through which racism shapes racial inequities in health. In addition to increasing awareness of systemic racism, this research will directly inform policy solutions. The following are calculations for select measures:
DISSIMILARITY INDEX
The formula used to calculate the dissimilarity index for two racial/ethnic groups within a geographic region is as follows:
Where P1i is the population of group 1 in the ith area (e.g. census tract), P2i is the population of group 2 in the ith area (e.g. census tract), P1 is the total population of group 1 in the geographic region for which the index of dissimilarity is being calculated (e.g. city), P2 is the total population of group 2 in the geographic region for which the index of dissimilarity is being calculated (e.g. city), and n is the number of spatial units (e.g. census tracts) (53).
The value D is interpreted as the proportion of a group that would need to move across spatial units in order to achieve equal distribution of the population. D ranges from 0 to 100. A value of 0 indicates total integration; 100 represents when each spatial unit contains only one group (total segregation).
ENTROPY INDEX
The formula used to calculate the entropy index, which assesses the spatial distribution of multiple groups simultaneously in a geographic region is as follows:
Where k is the number of racial/ethnic groups, pij is the proportion of population jth race/ethnicity in geographic region i (53).
The value h has a maximum value of ln(k). Higher values of h represent more equal distribution across racial/ethnic groups.
DIVERGENCE INDEX
The formula used to calculate the divergence index for each group in location i is the following:
Where the symbol pi is group m’s proportion of the population in location i, is group m’s proportion of the overall population (54). When the value Di = 0, then the location has the same composition as the overall population, indicating no segregation in the location. The overall segregation of a geographic region (e.g., city) is the population-weighted average of the divergence for all locations i (e.g., census tracts) and follows the following formula:
Where T is the overall population count in the geographic region, and t i is the population count for location i. When the value D = 0 then all locations have the same composition as the overall population in the region, indicating no segregation in the geographic region.
EXPOSURE AND ISOLATION INDICES
The formula used to calculate the exposure (or interaction) index between two groups within a geographic region is as follows:
Where P1i is the population of group 1 in location i, P1 is the population of group 1 in the geographic region, P2i is the population of group 2 in location i, Ti is the total population in location i, and n is the number of locations in the geographic region (53).
The formula for calculation of the isolation index for a group within a geographic region is as follows:
Where P1i, P1, T i, and n all denote the same as above (53).
The value of P ranges between 0 and 1, and can be interpreted in the case of the exposure index as the probability that a randomly chosen member of group 1 shares the same location with a member of group 2, and in the case of the isolation index, as the probability that a member of group 1 shares the same location with another member of group 1.
CONCENTRATION INDICES
There are three measures of concentration: delta, absolute concentration, and relative concentration. The formula for calculation of the delta index for a group in a geographic region is as follows:
Where xi represents the population of a group in location i, X represents the population of the group in the geographic region, ai equals the land area of location i, and A equals the total land area in the geographic region (53).
The formula for calculation of the absolute concentration for a group in a geographic region is as follows:
Where the geographic units (locations) are ordered by geographic size from smallest to largest, ai is the land area of location i, n1 and n2 equal the different points in the rank ordering of areal units from smallest to largest, with n1 representing the rank of the geographic unit where the cumulative total population of areal units equals the total minority population of the geographic region, summing from the smallest unit up; and n2 is the rank of the geographic unit where the cumulative total population of units equals the minority population totaling from the largest unit down (53). T1 equals the total population of geographic units from 1 to n1, T2 equals the total population of geographic units from n2 to n, ti refers to the total population of the location i, and X is the number of a group in the geographic region. X and xi, denote the same as above.
The formula for calculation of the relative concentration between two groups in a geographic region is as follows:
Where again geographic units are ordered from smallest to largest in areal size, and ai, n1, n2, ti, T1, and T2 are all the same as above. xi and yi represent the populations of each group in location i. X and Y represent the population of each group in the geographic region (53).
CLUSTERING
There are two measures of clustering: absolute clustering and relative clustering. The formula for calculating the index of absolute clustering is as follows:
Where xi represents the population of a group in location i, X represents the population of the group in the geographic region, xj equals the population of a group in location j, cij refers to an element in a “contiguity matrix” that equals one when geographic locations i and j are contiguous and zero otherwise, tj equals the total population of the location j, and n equals the areal units (53).
The formula for calculating the index of relative clustering is as follows:
Where xi and xj represent the population of group x in locations i and j, respectively, and yi and yj represent the population of group y in locations i and j, respectively. X and Y represent the population of groups X and Y in geographic region. cij is the same as above (53).
INDEX OF CONCENTRATION AT THE EXTREMES (ICE)
The formula for calculating the ICE between two groups in a geographic unit i:
Where Ai is the number of people belonging to most privileged extreme, Pi is the number of people belonging to the most deprived extreme, and Ti is the total population for which the privilege level was assessed (55).
ICE ranges from -1 to 1, where -1 denotes that 100% of the population in geographic unit i are in the most deprived group and 1 denotes that 100% of the population in geographic unit i are in the most privileged group.
COMPOSITE MEASURES OF SYSTEMIC RACISM
Creating a composite measure of systemic racism can also be achieved by examining these indices of racial inequity jointly. A composite approach is appropriate when researchers aim to capture an overall estimate of systemic racism, as this approach acknowledges that racism shows up in the mutually reinforcing and interconnected systems of society (4). For instance, in the United States, racial inequities in systems of employment, education, and incarceration are inextricably linked via laws and policies. The central tenants of systemic racism (i.e., multifaceted, interconnected, institutionalized, relational subordination/superordination that manifests in racial inequities in life chances) and their implications for measurement are elaborated by Brown and Homan (57). A composite approach also avoids problems with multicollinearity when multiple indicators of systemic racism are used as observed variables in the same model (see 58, Online Supplemental Material).
Initial health outcomes research utilized composite scores of systemic racism derived from the above indicators, with the goal being to use multiple indicators to approximate systemic racism in the domains of political participation, employment and job status, educational attainment, and judicial treatment (e.g., 48; 58). The relative proportion of racially minoritized individuals to white individuals on each indicator in a given geographic unit is first taken or derived from administrative data. This relative proportion is then dichotomized as “high” or “low” using a median cut point. Finally, dichotomized variables are summed or averaged for each geographic unit to calculate a composite score of systemic racism for that unit.
Research has advanced to model systemic racism as a latent variable. For instance, Dougherty et al. (59; Appendix) and Dougherty and Dean (60), use confirmatory factor analyses to first establish a parsimonious measure of systemic racism for their specific investigation. This approach minimizes the effect of random measurement error. It also provides an advantage over the simple calculation of sum or mean scores, which have traditionally relied on median splits and counts of the total number of indicators which makes missing data on some indicators for some geographic units a computational challenge.
Selection of indices of systemic racism used in a composite score or to test in a latent variable framework should be motivated by the specific research question and outcome of interest. Beyond the indices detailed above, sociology scholars of systemic racism Brown and Homan (57) provide a detailed account of systemic racism measurement and a repository of structural racism measures especially appropriate for health research (see article Appendix).
RACIAL BIAS IN MORTGAGE LENDING AND REDLINING INDICES
The Racial Bias in Mortgage Lending and Redlining Indices can be estimated using data from the publicly available Home Mortgage and Disclosure Act (HMDA) database and logistic regression models to estimate odds ratios. The Racial Bias in Mortgage Lending Index is calculated as the odds ratio for denial of a mortgage application from a Black applicant compared to denial of a White applicant (20). The Redlining Index is calculated as the odds ratio for denial of the mortgage application for individuals in one area compared to outside of that area (20). By using logistic regressions to calculate odds ratios, researchers can adjust for other factors such as sex and ratio of the loan amount to the applicant’s gross annual income.
There are two main spatial approaches to estimating these indices: estimation within specific units (e.g. census tract) or continuous estimation over a geographic region using the adaptive spatial filtering (ASF) approach. Estimation within specific units will create an estimate for each geographic unit. Integration of logistic regression with ASF will create a continuous surface for the statistic of interest across the geographic region (56).
Author(s) and Reviewer(s): Prepared by David H. Chae, Sc.D., Connor D. Martz, M.S., Kara W. Chung, M.S., Erica B. Doe, M.S.W., Diamond J. Cunningham, M.P.H., Emma A. Blackson, M.P.H., Vanessa Volpe, Ph.D., Olivenne Skinner, Ph.D., and Thomas A. LaVeist, Ph.D. Reviewed by Julian Thayer, Ph.D., Mark Hatzenbuehler, Ph.D., Agus Surachman, Ph.D., and Elissa Epel, Ph.D..
References
-
J. Feagin, Z. Bennefield, Systemic racism and U.S. health care. Soc. Sci. Med. 103, 7–14 (2014).
-
N. Krieger, Discrimination and Health Inequities. Int. J. Health Serv. 44, 643–710 (2014).
-
A. A. Sewell, The Racism-Race Reification Process: A Mesolevel Political Economic Framework for Understanding Racial Health Disparities. Sociol. Race Ethn. 2, 402–432 (2016).
-
Z. D. Bailey, et al., Structural racism and health inequities in the USA: Evidence and interventions. Lancet 389, 1453–1463 (2017).
-
C. C. Gravlee, Systemic racism, chronic health inequities, and COVID‐19: A syndemic in the making? Am. J. Hum. Biol., e23482 (2020).
-
K. Ture, C. V. Hamilton, S. Carmichael, Black power: The politics of liberation in America (Vintage, 1992).
-
Y. C. Paradies, Defining, conceptualizing and characterizing racism in health research. Crit. Public Health 16, 143–157 (2006).
-
J. Feagin, Systemic racism: A theory of oppression (Routledge, 2013).
-
E. Bonilla-Silva, Rethinking Racism: Toward a Structural Interpretation. Am. Sociol. Rev. 62, 465–480 (1997).
-
R. Rothstein, The color of law: A forgotten history of how our government segregated America (Liveright Publishing, 2017).
-
N. Krieger, et al., Structural Racism, Historical Redlining, and Risk of Preterm Birth in New York City, 2013-2017. Am. J. Public Health 110, 1046–1053 (2020).
-
E. E. Lynch, et al., The legacy of structural racism: Associations between historic redlining, current mortgage lending, and health. SSM - Popul. Health 14, 100793 (2021).
-
M. R. Kramer, “Residential Segregation and Health” in Neighborhoods and Health, 2nd Ed., (Oxford University Press, 2018) https:/doi.org/10.1093/oso/9780190843496.003.0012 (September 23, 2021).
-
N. Krieger, et al., Public Health Monitoring of Privilege and Deprivation With the Index of Concentration at the Extremes. Am. J. Public Health 106, 256–263 (2015).
-
E. Gelormino, G. Melis, C. Marietta, G. Costa, From built environment to health inequalities: An explanatory framework based on evidence. Prev. Med. Rep. 2, 737–745 (2015).
-
C. E. Ross, J. Mirowsky, Disorder and Decay: The Concept and Measurement of Perceived Neighborhood Disorder. Urban Aff. Rev. 34, 412–432 (1999).
-
B. Woo, et al., Residential Segregation and Racial/Ethnic Disparities in Ambient Air Pollution. Race Soc. Probl. 11, 60–67 (2019).
-
J. D. Kaufman, A. Hajat, Confronting Environmental Racism. Environ. Health Perspect. 129, 051001 (2021).
-
Federal Financial Institutions Examination Council (FFIEC), HMDA Data Browser (September 22, 2021).
-
Y. Zhou, A. Bemanian, K. M. M. Beyer, Housing Discrimination, Residential Racial Segregation, and Colorectal Cancer Survival in Southeastern Wisconsin. Cancer Epidemiol. Prev. Biomark. 26, 561–568 (2017).
-
D. D. Mendez, V. K. Hogan, J. Culhane, Institutional Racism and Pregnancy Health: Using Home Mortgage Disclosure Act Data to Develop an Index for Mortgage Discrimination at the Community Level. Public Health Rep. 126, 102–114 (2011).
-
R. DeAngelis, Systemic Racism in Police Killings: New Evidence from the Mapping Police Violence Database, 2013-2021. Race Justice (2021) https:/doi.org/10.1177/21533687211047943.
-
S. Sinyangwe, D. McKesson, J. Elzie, Mapping Police Violence Database. Mapp. Police Violence Database (September 22, 2021).
-
B. Burghart, Methodology – Fatal Encounters (2019) (September 22, 2021).
-
The Counted: people killed by police in the United States – interactive | US news | The Guardian (September 22, 2021).
-
Fatal Force: Police shootings database. Wash. Post (September 22, 2021).
-
A. A. Sewell, K. A. Jefferson, H. Lee, Living under surveillance: Gender, psychological distress, and stop-question-and-frisk policing in New York City. Soc. Sci. Med. 1982 159, 1–13 (2016).
-
J. G. Alson, W. R. Robinson, L. Pittman, K. M. Doll, Incorporating Measures of Structural Racism into Population Studies of Reproductive Health in the United States: A Narrative Review. Health Equity 5, 49–58 (2021).
-
C. Wildeman, E. A. Wang, Mass incarceration, public health, and widening inequality in the USA. The Lancet 389, 1464–1474 (2017).
-
J. Schnittker, C. Uggen, S. K. S. Shannon, S. M. McElrath, The Institutional Effects of Incarceration: Spillovers From Criminal Justice to Health Care. Milbank Q. 93, 516–560 (2015).
-
R. C. Johnson, S. Raphael, The Effects of Male Incarceration Dynamics on Acquired Immune Deficiency Syndrome Infection Rates among African American Women and Men. J. Law Econ. 52, 251–293 (2009).
-
M. Mauer, R. S. King, “Uneven justice: State rates of incarceration by race and ethnicity” (The Sentencing Project, 2007) (September 22, 2021).
-
Vera Institute of Justice, Incarceration Rates - Incarceration Trends - Vera Institute of Justice (September 22, 2021).
-
M. L. Hatzenbuehler, K. Keyes, A. Hamilton, M. Uddin, S. Galea, The Collateral Damage of Mass Incarceration: Risk of Psychiatric Morbidity Among Nonincarcerated Residents of High-Incarceration Neighborhoods. Am. J. Public Health 105, 138–143 (2015).
-
Execution Database. Death Penal. Inf. Cent. (October 7, 2021).
-
Capital Punishment. Bur. Justice Stat. (October 7, 2021).
-
D. Pager, B. Western, Identifying Discrimination at Work: The Use of Field Experiments. J. Soc. Issues 68, 221–237 (2012).
-
P. M. Kline, E. K. Rose, C. R. Walters, “Systemic Discrimination Among Large U.S. Employers” (National Bureau of Economic Research, 2021) https:/doi.org/10.3386/w29053 (September 22, 2021).
-
EEOC Releases Fiscal Year 2017 Enforcement and Litigation Data | U.S. Equal Employment Opportunity Commission (September 22, 2021).
-
Injustice at Work (Center for Public Integrity, 2021) (September 22, 2021).
-
US Census Bureau, American Community Survey 5-Year Data (2009-2019). U. S. Census Bur. (October 7, 2021).
-
National Center for Health Statistics, NAMCS/NHAMCS - About the Ambulatory Health Care Surveys (2019) (September 23, 2021).
-
K. N. Ray, A. V. Chari, J. Engberg, M. Bertolet, A. Mehrotra, Disparities in Time Spent Seeking Medical Care in the United States. JAMA Intern. Med. 175, 1983–1986 (2015).
-
A. K. Mathur, et al., Variation in access to the liver transplant waiting list in the United States. Transplantation 98, 94–99 (2014).
-
J. M. Wisniewski, B. Walker, Association of Simulated Patient Race/Ethnicity With Scheduling of Primary Care Appointments. JAMA Netw. Open 3, e1920010 (2020).
-
H. Kugelmass, “Sorry, I’m Not Accepting New Patients”: An Audit Study of Access to Mental Health Care. J. Health Soc. Behav. 57, 168–183 (2016).
-
Z. Obermeyer, B. Powers, C. Vogeli, S. Mullainathan, Dissecting racial bias in an algorithm used to manage the health of populations. Science 366, 447–453 (2019).
-
A. Lukachko, M. L. Hatzenbuehler, K. M. Keyes, Structural racism and myocardial infarction in the United States. Soc. Sci. Med. 1982 103, 42–50 (2014).
-
US Census Bureau, Voting and Registration. U. S. Census Bur. (October 7, 2021).
-
National Conference of State Legislatures, State Legislator Demographics (2020) (October 7, 2021).
-
J. Kavanagh, C. B. Gibson, S. Cherney, “Database of State Voting Laws: Preparing for Elections During a Pandemic” (RAND Corporation, 2020) (October 7, 2021).
-
S. Ansolabehere, B. F. Schaffner, CCES Common Content, 2016. CCES16_Common_OUTPUT_Feb2018_VV.tab (2017) https:/doi.org/10.7910/DVN/GDF6Z0/JPMOZZ.
-
D. S. Massey, N. A. Denton, The Dimensions of Residential Segregation. Social Forces 67, 281–315 (1988).
-
E. Roberto, The Divergence Index: A Decomposable Measure of Segregation and Inequality. arXiv:1508.01167, 1–26 (2016).
-
N. Krieger, R. Kim, J. Feldman, P. D. Waterman, Using the Index of Concentration at the Extremes at multiple geographical levels to monitor health inequities in an era of growing spatial social polarization: Massachusetts, USA (2010–14). International Journal of Epidemiology 47, 788–819 (2018).
-
K. M. M. Beyer, et al., New spatially continuous indices of redlining and racial bias in mortgage lending: links to survival after breast cancer diagnosis and implications for health disparities research. Health & Place 40, 34–43 (2016).
-
T. H. Brown, & P. Homan. Structural racism and health stratification: Connecting theory to measurement. J Health Social Behav, 65(1), 141-160. (2024). https://doi.org/10.1177/00221465231222924
-
V. V. Volpe, et al. State- and provider-level racism and health care in the U.S. Am J Prev Med, 61(3), 338-347. (2021). https://doi.org/10.1016/j.amepre.2021.03.008
-
G. B. Dougherty, et al. Measuring structural racism and its association with BMI. Am J Prev Med, 59(4), 530-537. (2020). https://doi.org/10.1016/j.amepre.2020.05.019
-
G. B. Dougherty, & L. Dean. Using theory to define a computationally tractable specification space in confirmatory factor modeling. 2017 Stata Conference 16, Stata Users Group. https://ideas.repec.org/p/boc/scon17/16.html


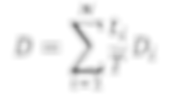






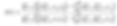

